Research Interests
My main occupation is the development of Machine Learning methodologies aimed at solving particular problems other disciplines might present. In particular, I have extensively worked with both simulated and observed astronomical data sets. In the first case, I have contributed significantly to development of the public toolbox 1-DREAM. We present here 5 methodologies that gradually discover one-dimensional structures (filaments, streams, etc.). For each detected filament we recover an explicit parametric formulation, as a mapping from a latent abstract graph to its embedding in the data space. Thanks to this smooth mapping, we are able to walk along the tangent space of the streams and study their physical properties. At the same time, taking advantage of the probabilistic modelling over their skeleton, we can produce maps on the co-moving orthonormal coordinate frames along the manifold. This allows for detailed studies of the dynamics and hcemical properties of the filaments. The same methodology has been applied to both simulations of Jellyfish galaxies and the Large-Scale-Structure of the Universe (Cosmic Web).
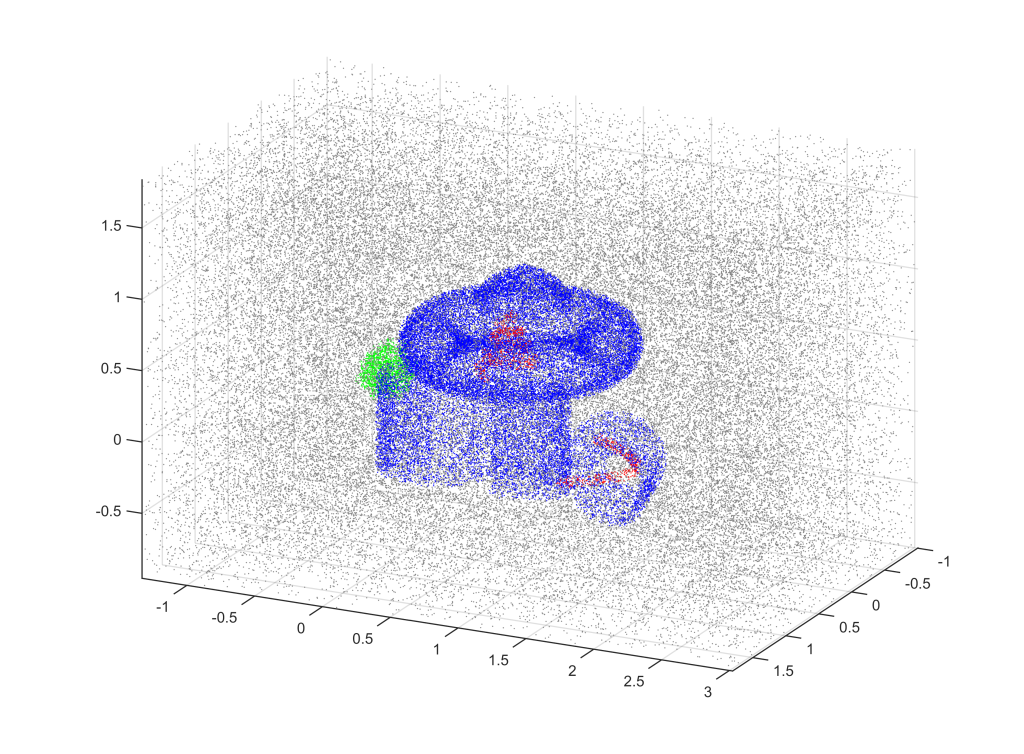
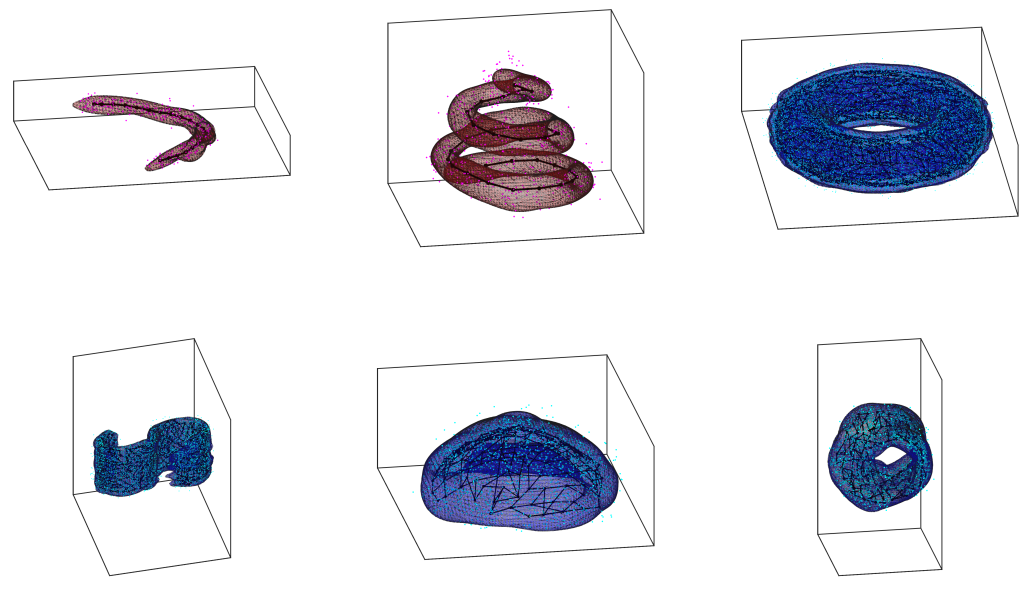
During my years as a Research Fellow at UoB I have also worked on biomedical problems. In particular, multiple works have been performed on the study of metabolomic pathways in the early diagnosis of Cushing disease. Further studies on feature relevance in the distinction between Non-fat Adrenocortical Tumors (NFAT) and Mild-Autonomous-Cortisol-Secretion (MACS) are on the way and soon to be submitted.
I also had the chance to colaborate with epydemiologists at the Institute of Applied Health Research (@UoB) within the OPTIMAL project. The focus is here to describe multi-morbidity and develop a toolbox for clinicians and researches capable of guiding epidemiological studies on different sub-populations, distinct by demography. An additional project focuses on poli-pharmacy and how to prevent over-exposure to medications to patients with multiple long term cronic diseases.
Publications
- M. Canducci, P. Tiño, M. Mastropietro,Probabilistic modelling of general noisy multi-manifold data sets, Artificial Intelligence, Volume 302, 2022, 103579, ISSN 0004-3702.
- M. Canducci et al., Tracking the Temporal-Evolution of Supernova Bubbles in Numerical Simulations. In: Yin, H., et al. Intelligent Data Engineering and Automated Learning – IDEAL 2021. IDEAL 2021. Lecture Notes in Computer Science(), vol 13113. Springer, Cham.
- A. Taghribi, M. Canducci, M. Mastropietro, S. De Rijcke, K. Bunte, P. Tiňo, ASAP – A sub-sampling approach for preserving topological structures modeled with geodesic topographic mapping, Neurocomputing, Volume 470, 2022, Pages 376-388, ISSN 0925-2312,
- M. Canducci, P. Awad, A. Taghribi, M. Mohammadi, M. Mastropietro, S. De Rijcke, R. Peletier, R. Smith, K. Bunte, P. Tiňo, 1-DREAM: 1D Recovery, Extraction and Analysis of Manifolds in noisy environments, Astronomy and Computing, Volume 41, 2022, 100658,ISSN 2213-1337.
- Petra Awad, Reynier Peletier, Marco Canducci, Rory Smith, Abolfazl Taghribi, Mohammad Mohammadi, Jihye Shin, Peter Tiňo, Kerstin Bunte, Swarm-intelligence-based extraction and manifold crawling along the Large-Scale Structure, Monthly Notices of the Royal Astronomical Society, Volume 520, Issue 3, April 2023, Pages 4517–4539.